
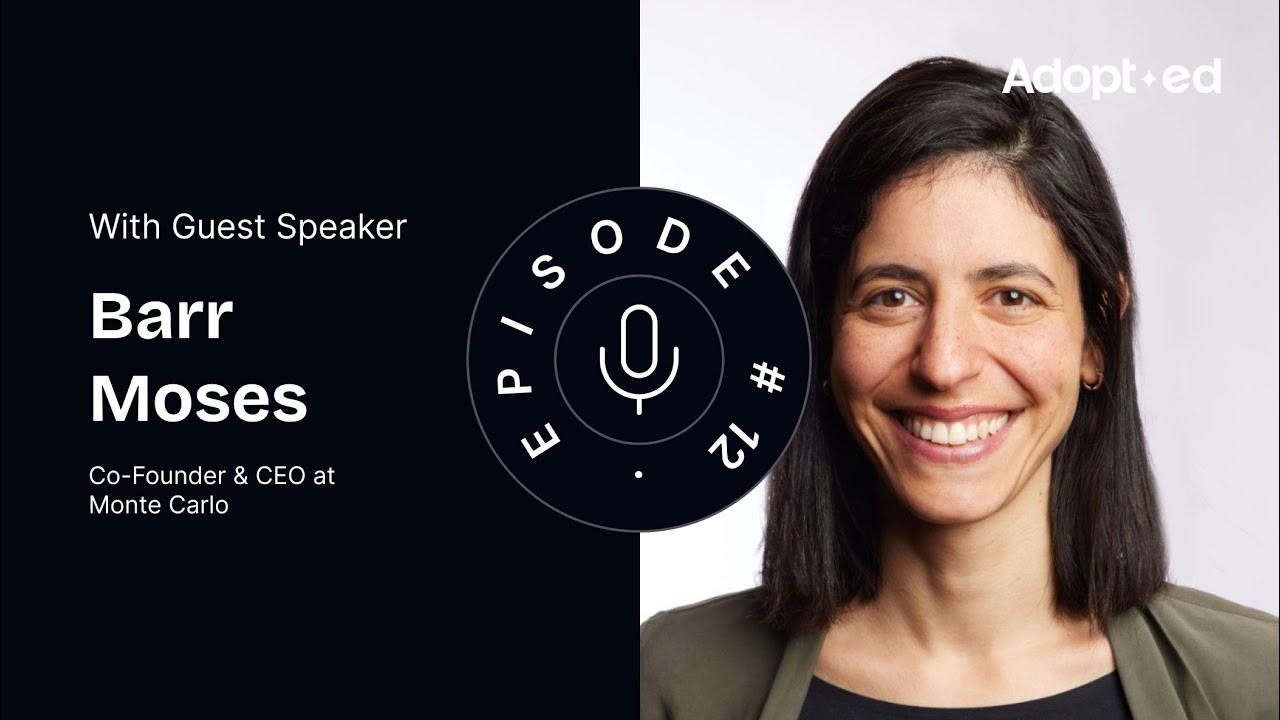
I recently had an illuminating conversation with Barr Moses, CEO of Monte Carlo and pioneer in data observability.
If you work in this industry, you already know there's a pivotal shift underway - we're rapidly moving from systems that merely provide information to those that actively execute tasks on our behalf.
And while the transition from "here's what you should know" to "I'll handle this for you" represents a quantum leap in AI capabilities, it also dramatically raises the stakes for data quality.
Barr Moses explained in our recent conversation:
"The implications of data being wrong five to ten years ago were maybe minimal. Maybe our CEO was kind of looking at the data and was a little bit upset that the data was wrong. But today, the stakes are a lot higher."
The rules have fundamentally changed, and organizations need to adapt quickly. Read ahead to learn more insights from our conversation.
The Four Pillars of AI Failure
During our conversation, Barr outlined four fundamental causes of AI system failures that every organization implementing AI agents should understand:
"We largely find that there are four core reasons for why your data and AI estate might have problems.
It can be traced to four core pillars.
The first is if you have bad data.
The second core reason is change in your code.
The third thing that can go wrong is you might have a problem with the system or the infrastructure.
And then the fourth thing that can go wrong is the model response that you have might be unfit for the use case."
Let's explore each of these pillars, as they form the foundation for understanding both the risks and opportunities in actionable AI.
1. Bad Data: The Root of AI Failures
The most common source of AI failures is simply bad data. This includes incomplete, outdated, biased, or misrepresented information that leads to flawed decision-making.
Bad data manifests in numerous ways that can wreak havoc on AI systems, some examples -
- Missing values in customer profiles leading to incorrect personalization
- Duplicate records causing double-counting in inventory systems
- Inconsistent date formats triggering erroneous time-based actions
- Stale data that no longer reflects current business conditions
A recent Monte Carlo survey confirms the scope of this problem—a staggering 66% of data teams experienced a data incident costing their organization $100,000 or more in the past 6 months alone.

.
2. Code Changes: The Invisible Disruptor
The second pillar focuses on how changes to code - whether in data transformations or the AI models themselves, can cause failures. Code changes that seem innocuous can profoundly impact how data is processed and understood by AI agents.
Even seemingly minor code modifications can have far-reaching consequences:
- A simple change in sort order (ASC vs DESC) completely inverting prioritization logic
- Updated SQL join conditions silently excluding valid records
- Parameter changes in ML models that shift decision boundaries
- New data transformations that handle null values differently than before
- Library or dependency updates that subtly alter algorithmic behavior
According to the survey, 54% of teams still rely on manual testing or have no initiative in place to address these quality issues in their AI systems.
This means that code changes often go undetected until they've already caused significant problems.
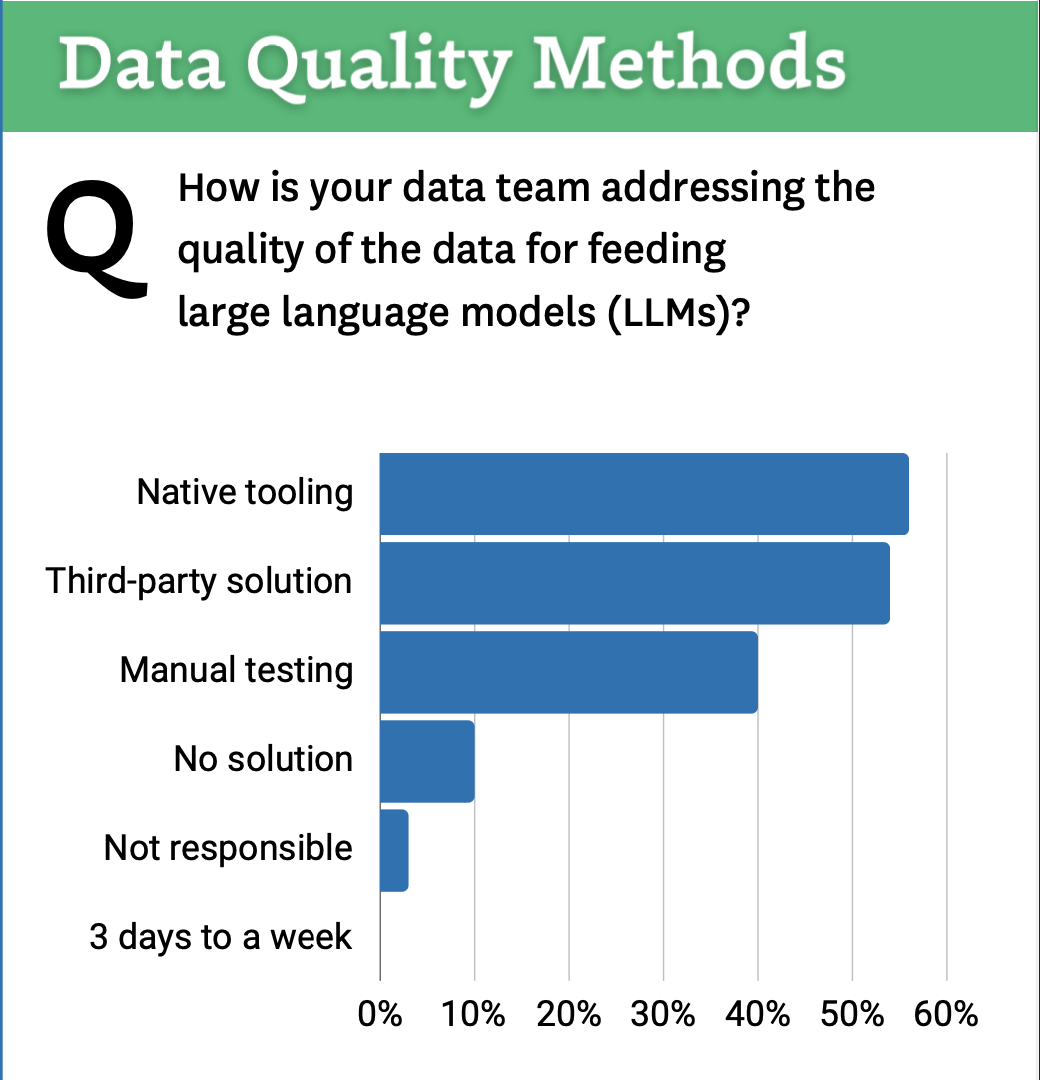
3. System and Infrastructure Issues: The Technical Foundation
The third pillar encompasses failures in the systems and infrastructure that power AI operations—failed jobs, overloaded servers, networking issues, and other technical breakdowns.
These issues are particularly insidious because they can be intermittent and difficult to reproduce.
4. Unfit Model Responses: The AI's Understanding Gap
The final pillar involves situations where, even with perfect data, code, and infrastructure, the model's response is simply not appropriate for the given context or use case.
This manifests as hallucinations, inappropriate suggestions, or actions that don't align with business logic or ethics.
As Barr pointed out:
"The model response that you have might be unfit for the use case. So you can have the perfect prompt and the perfect contents, and still the model response would be inappropriate or not fit... like the Google and the Super Glue example."
This refers to an incident where someone asked Google what to do if cheese is slipping off pizza, and the AI suggested using super glue—a response that was clearly inappropriate despite everything else working correctly.
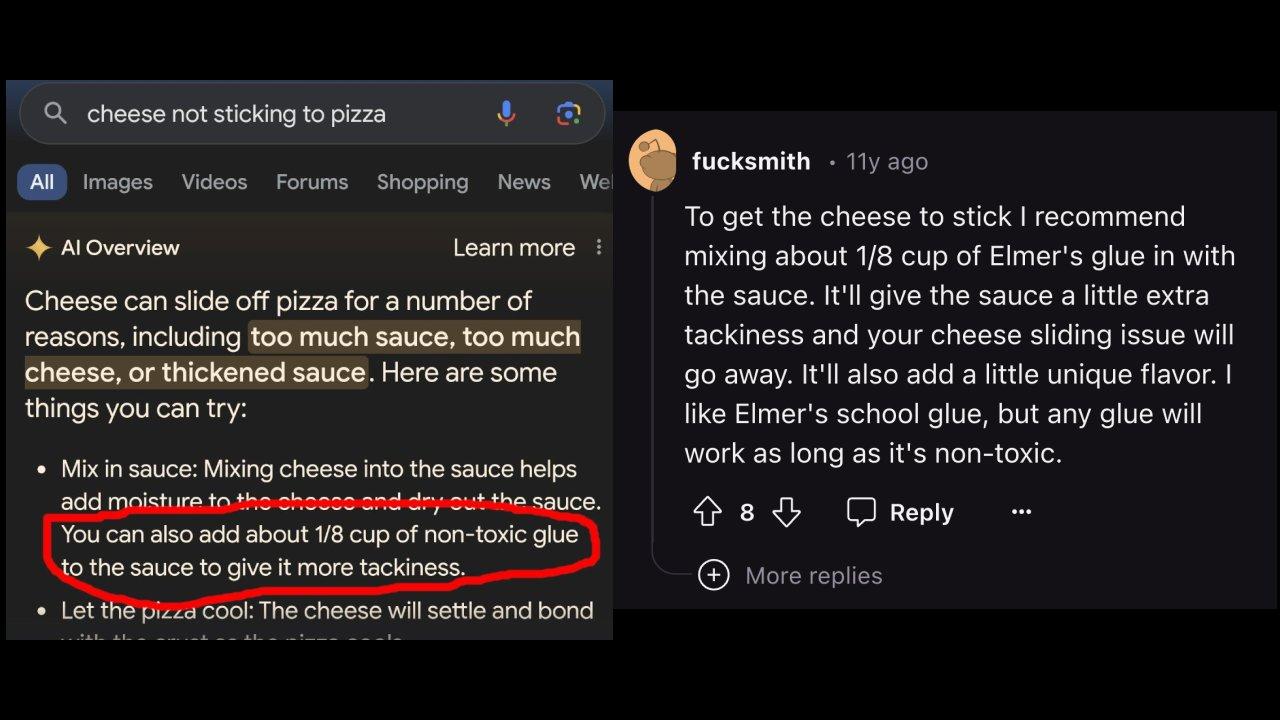
Real-World Impact: When Data Quality Fails
Barr shared several sobering examples that illustrate just how devastating data quality failures can be:
- A gaming company (Unity) suffered a $100 million loss and a 37% stock drop due to a single schema change that corrupted their advertising algorithm.
- Citibank faced a $400 million regulatory fine for inadequate data governance practices
- An automotive retailer's AI agent was manipulated to sell a Chevy Tahoe for $1, exposing a fundamental vulnerability in their business model
These examples highlight how the shift to agentic AI fundamentally transforms risk profiles.
This new paradigm introduces several critical vulnerabilities:
Irreversible Actions: Many agent actions - financial transactions, contract commitments, resource allocations - cannot be easily reversed. For example, once an AI transfers funds or makes promises to customers, undoing these actions is technically challenging and also erodes trust and potentially also creates legal liability.
Cascading Effects: In interconnected enterprise systems, a single action based on bad data can trigger chain reactions across multiple systems.
Timing Criticality: Many agent-driven actions are time-sensitive, leaving little opportunity for human review before execution. The value of an autonomous agent diminishes if every action requires human approval, creating pressure to reduce oversight precisely when it may be most needed.
Attribution Complexity: When something goes wrong, determining whether the issue stemmed from data, code, systems, or model limitations becomes increasingly difficult. This complexity makes prevention even more important than forensics.
The Model Commoditization Inflection Point
When contemplating data quality, there's another crucial dimension that dramatically amplifies its importance: the rapid commoditization of AI models themselves.
As Barr Moses insightfully explains:
"For enterprises, the thing to remember is that anyone has access to the latest and greatest model... within a few seconds or a couple of minutes of like setting it up, I can, right, like get the API key and we're off to the races. And so how do enterprises actually differentiate in that world? The value is in the enterprise data."
This commoditization creates a fascinating paradox: as models become more powerful, they also become more interchangeable.
Your competitive advantage no longer comes from which model you use, but from what you feed it.
This explains why industries with the highest stakes - healthcare, finance, manufacturing, are leading investments in data quality infrastructure rather than simply chasing the latest model releases.
Conclusion: Navigating the New Data Quality Imperative
As we journey deeper into this agentic AI revolution, the relationship between data and models is undergoing a fundamental inversion.
In the previous era, we focused on finding models sophisticated enough to extract value from our data. Now, we're racing to ensure our data is sophisticated enough to extract value from increasingly commoditized models.
This shift is rewriting the rules of software as we know them.
And perhaps that's the most exciting part of this journey. We're witnessing not just a technological evolution, but a fundamental reimagining of how software, data, and human needs intersect.
In this new landscape, one thing remains certain: the path to exceptional AI runs directly through exceptional data.